What Are Pre-Trained Multi-Task Generative AI Models?
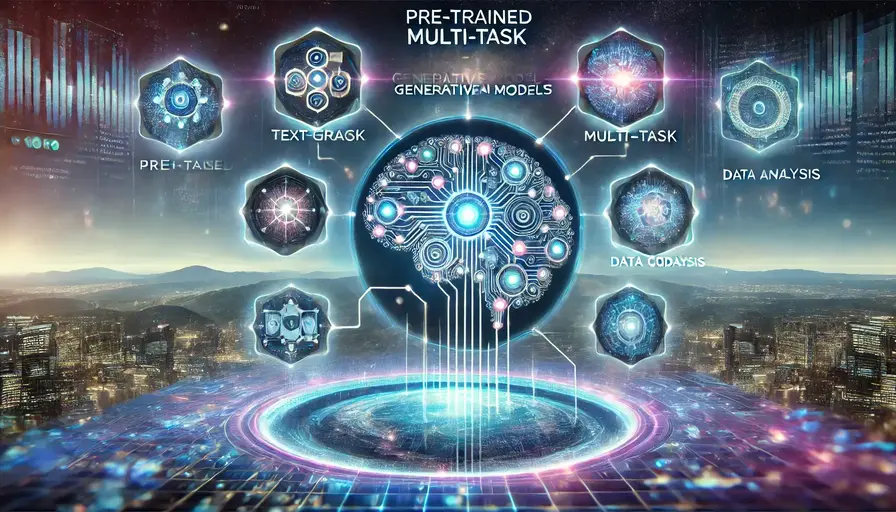
Artificial intelligence (AI) is advancing at an unprecedented tempo, and one in each of its most transformative dispositions is the emergence of Pre-Trained Multi-Task Generative AI Models, moreover referred to as foundation models. These models have the ability to address a number of tasks, from answering multiple- choice questions (MCQs) to generating innovative content material like poetry or even supporting in software development.
In this article, we’ll discover what are pre-trained multi-task generative AI models are called, and how they work, their real-world packages, and why they’re often defined as “brains” of AI systems. We’ll additionally address associated phrases like “Pre-trained multi-task generative AI models are called MCQ”, “Pre-trained multi-task generative AI models are called Brain”, and “Pre-trained multi-task generative AI models are called Quizlet” to beautify your know-how/understanding.
Connecting Generative AI Models and Quantum Machine Learning
The global of AI is large, and technologies like Pre-Trained Multi-Task Generative AI Models and Quantum Machine Learning (QML) are making groundbreaking adjustments in how machines research and work/function. While generative AI models depend upon significant pre-education and deep getting to know strategies to address multiple responsibilities like textual content generation or coding, Quantum Machine Learning makes use of the unique properties of quantum computing to manner and examines statistics a good deal quicker. Imagine combining the power of generative models with the rate of QML—this may unlock even more opportunities, like generating insights from huge datasets in seconds or fixing fairly complicated troubles throughout industries. Together, those technologies are pushing the limits of what AI can achieve, making obligations smarter, faster, and more green.
What Are Pre-Trained Multi-Task Generative AI Models Are Called?
What are Pre-Trained Multi-Task Generative AI Models – are powerful AI systems skilled on large datasets to perform a huge variety of duties without requiring substantial retraining for every venture. These models are foundational, which means they serve as a base for specialized programs like textual content summarization, language translation, photo generation, and even coding.
For example, OpenAI’s GPT-4 is a much famous instance of such a model. It can help with writing essays, translating languages, creating summaries, and generating innovative content—all inside a single framework.
These models are frequently referred to as “foundation models” due to the fact they offer a solid base for building superior AI programs. To learn more, explore foundation models.
Pre-Trained Multi-Task Generative AI Models Are Called MCQ
The phrase “Pre-trained multi-task generative AI models are called MCQ” refers to the ability of these models to excel at answering multiple-choice questions (MCQs).
- These models analyze a query, understand its context, and examine multiple possible answers to select the exceptional one.
- Educators and e-gaining knowledge of structures use AI-powered gear to generate and grade MCQs, saving time and improving studying consequences.
For instance, high-quality-tuned variations of GPT models are used in training to routinely create MCQ quizzes tailored to particular mastering targets.
Pre-Trained Multi-Task Generative AI Models Are Called Brain
The term “Brain” aptly describes these models because of their ability to mimic human-like reasoning and adaptability. Just like a brain:
- They process and analyze information through interconnected layers of neural networks.
- They can adapt to a wide variety of tasks, such as generating text, analyzing data, or answering questions.
An example of this adaptability is Google Bard, which is designed to assist with everything from casual conversation to detailed research. This brain-like functionality makes these models integral to the future of AI.
Pre-Trained Multi-Task Generative AI Models Are Called Quizlet
The connection between these models and platforms like Quizlet lies in their ability to enhance personalized learning:
- They can create flashcards, quizzes, and study sets similar to what Quizlet offers.
- By analyzing user performance, they suggest areas for improvement, making learning more efficient.
For example, educators can fine-tune generative AI models to design tailored learning pathways for students, providing a Quizlet-like experience with greater customization.
How Pre-Trained Multi-Task Generative AI Models Work
In this article “what are pre-trained multi-task generative AI models” work. Let’s break down the functionality of Pre-Trained Multi-Task Generative AI Models into three steps:
1. Pre-Training
- The model is trained on massive datasets like books, articles, and websites.
- Using transformer architectures, it learns patterns, grammar, logic, and relationships within the data.
2. Multi-Task Learning
- These models are capable of performing multiple tasks simultaneously, such as summarizing text, generating code, or answering questions.
3. Fine-Tuning
- Developers fine-tune the model using smaller, task-specific datasets to customize it for particular applications, such as healthcare diagnostics or financial analysis.
For more details, explore how pre-trained models function.
Applications of Pre-Trained Multi-Task Generative AI Models
1. Healthcare
- Assists in generating diagnostic reports and analyzing patient data.
- Fine-tuned AI tools are used in telemedicine and disease prediction.
2. Content Creation
- Writers and marketers use these models to craft blogs, ad copy, and social media content.
- Jasper AI is an example of a generative AI device for marketers.
3. Education
- AI-powered tutoring structures provide personalized lessons and automated grading.
4. Software Development
- Developers use these models for code generation, debugging, and automation.
Check out real-world AI applications for more use cases.
Key Features of Pre-Trained Multi-Task Generative AI Models
1. Pre-Trained on Large Datasets
They learn from diverse datasets, making them highly versatile and knowledge-rich.
2. Generative Capabilities
These models can create new content, whether it’s text, code, or images.
3. Multi-Task Functionality
Their ability to handle diverse tasks with minimal retraining makes them highly efficient.
4. Efficiency
Fine-tuning is resource-friendly compared to building task-specific models from scratch.
For more insights, explore foundation model features.
Challenges and Ethical Considerations
Challenges
- Bias in Training Data: If training datasets are biased, models may produce skewed outputs.
- High Resource Costs: Training these models requires significant computational power.
- Potential Misuse: They could be exploited to create deep fakes or spread misinformation.
Ethical Considerations
- Transparent development and ethical guidelines are essential for their responsible use.
- Developers must address bias and ensure fairness in AI applications.
Explore AI ethics and challenges.
Why Should You Care About These Models?
Whether you’re a developer, commercial enterprise leader, or curious tech fanatic, Pre-Trained Multi-Task Generative AI Models are reshaping industries. They shop time, enhance creativity, and open new opportunities across healthcare, schooling, and past.
Conclusion
What are Pre-Trained Multi-Task Generative AI Models and how they are converting the manner we have interaction with generation. With their capability to evolve, generate, and perform multiple responsibilities, they constitute a brand new frontier in artificial intelligence.
As those basis models evolve, they promise to unlock unheard of possibilities, making era smarter, quicker, and greater intuitive. However, responsible development and ethical use are keys to harnessing their full potential.